Emergency Departments (EDs) are essential for providing prompt care but often face overcrowding issues, mainly due to non-urgent patients and seasonal spikes. Accurate forecasting of patient influx is essential for optimizing resource allocation and improving patient flow.
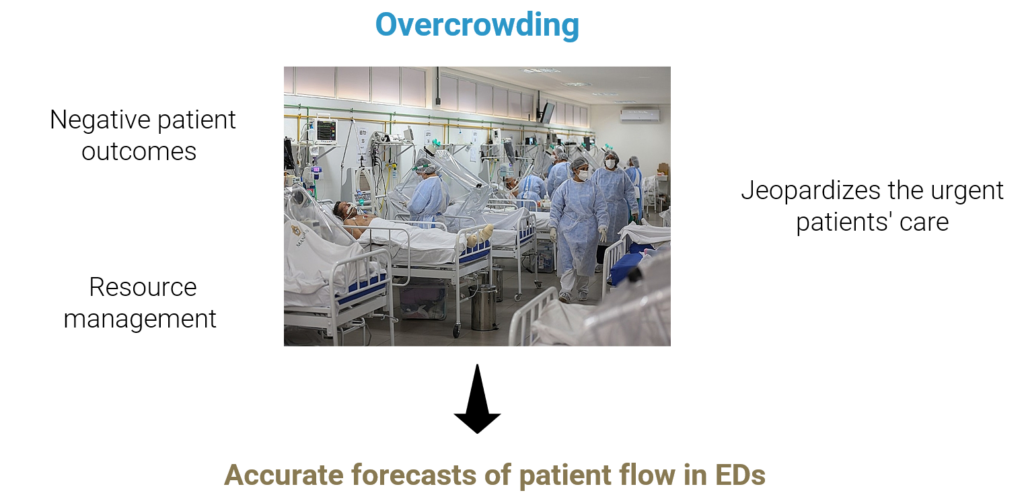
This work introduces the Hierarchical Temporal Disaggregator (HTD), a deep-learning hierarchical model that utilizes calendar and time-series covariates to predict daily patient numbers across different Regional Health Areas (RHAs), hospitals, and nationally.
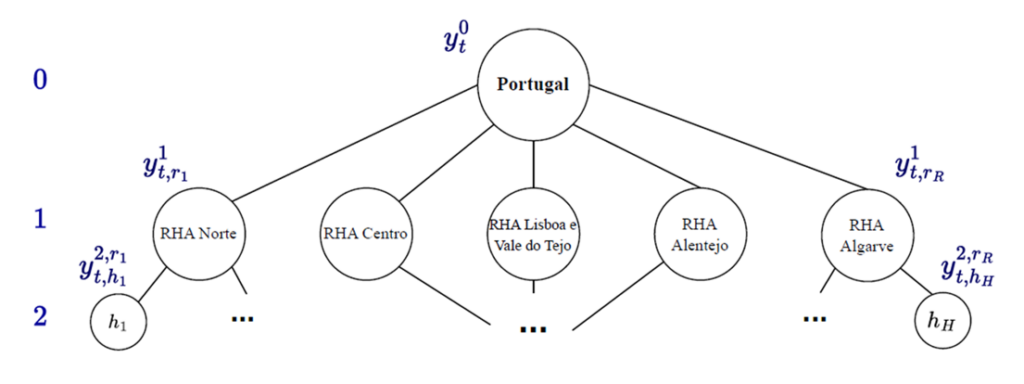
Our model directly generates accurate and reconciled forecasts for the entire hierarchy by effectively integrating both time-series data and explanatory variables.
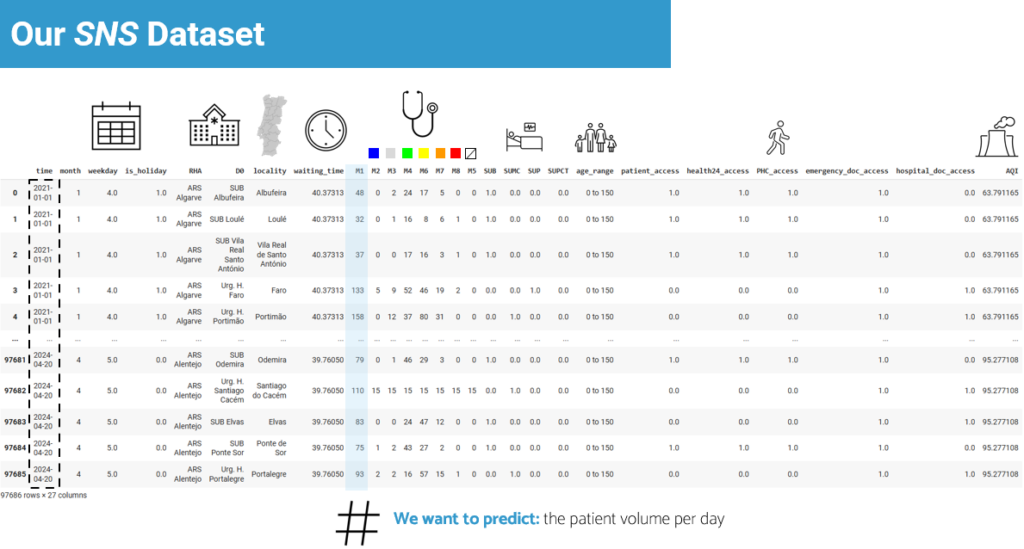
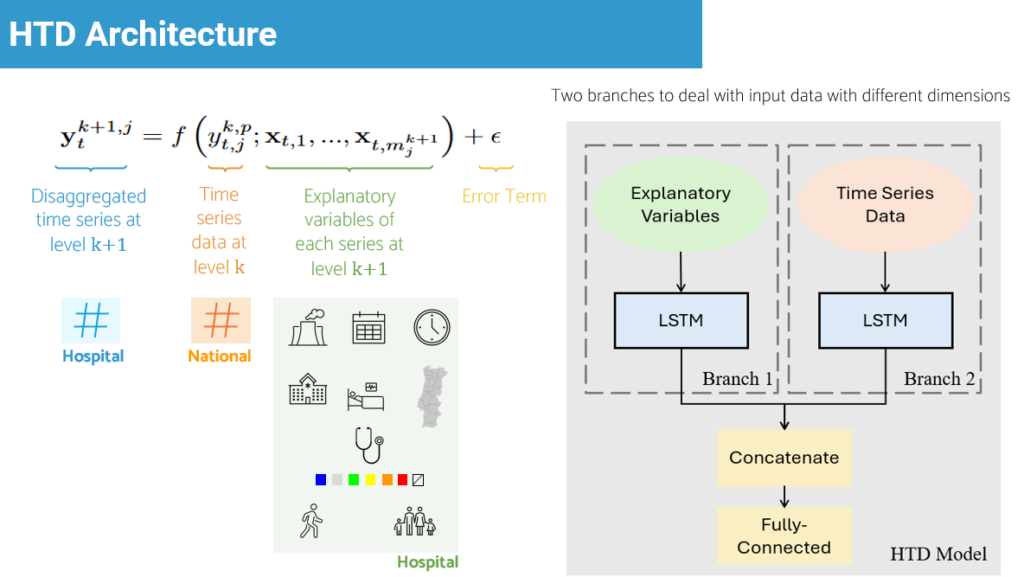
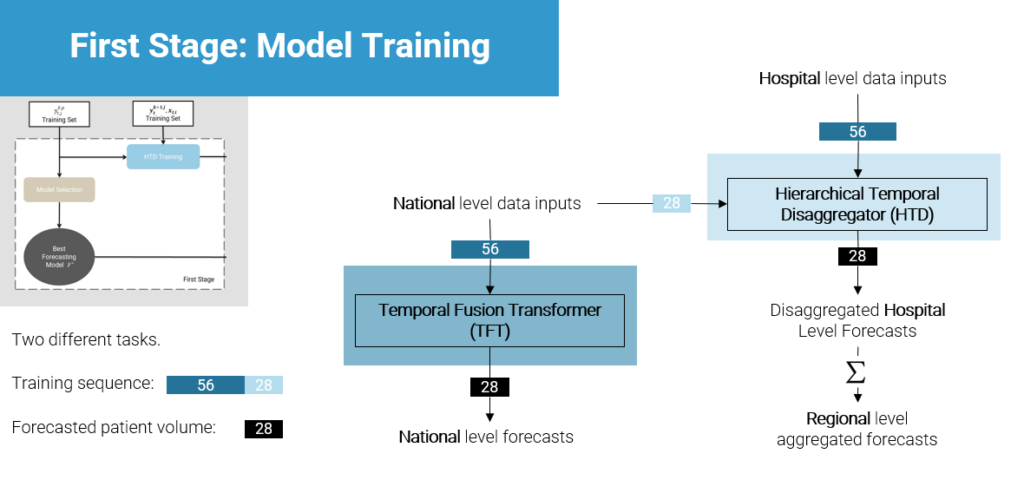
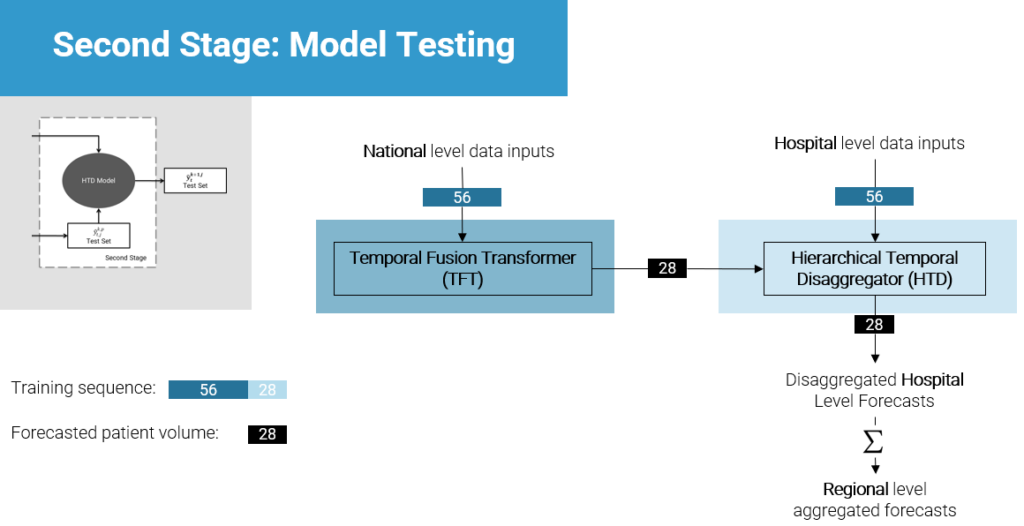
Experiments on the Portuguese National Health Service dataset demonstrate the superiority of HTD over conventional statistical and deep-learning models as well as standard hierarchical forecasting approaches. The HTD model achieved a Mean Absolute Percentage Error of 8.24% at the national level, 9.97% at the RHA level, and 16.55% at the hospital level, confirming its effectiveness and the added value of incorporating hierarchical and multivariate contexts in forecasting.
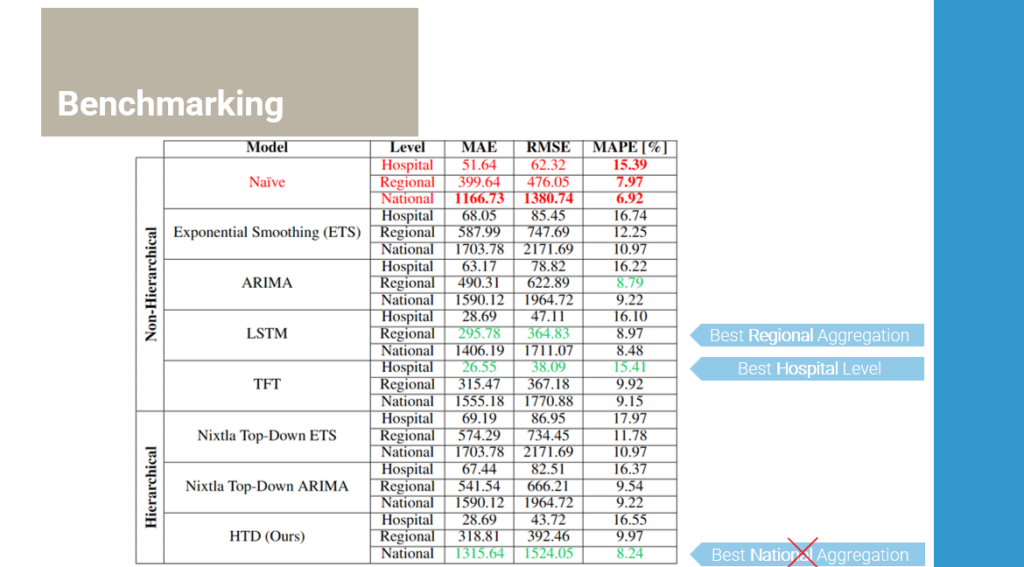